Machine learning: innovative technology within supply chains
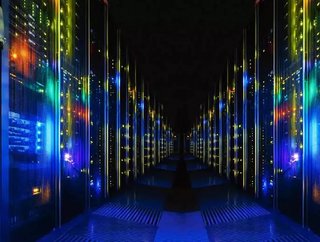
Watson is IBM’s suite of artificial intelligence (AI) services, applications and tools. Watson aims to help businesses unlock the value of data in new ways and remove repetitive tasks from employees to shift the focus to high-value work. This is in addition to allowing companies to predict and shape business outcomes in order to rethink practices and workflow.
A part of IBM Watson’s AI services is machine learning. Watson’s software has been developed to help data scientists and developers integrate AI into company applications. Watson Machine Learning enables cross-functional teams to deploy, monitor and optimise models quickly and easily.
BASF: Building a smart supply chain
BASF Nutrition and Health – a division of chemical group BASF – partnered with IBM in early 2019 as part of its wider vision to make digitalisation an integral part of the business.
BASF requires high delivery performance for its products with end-to-end coordination across suppliers, manufacturers, logistics partners and distribution centres as well as the ability to manage dysfunctional inventory. Partnering with IBM, BASF explored how AI and machine learning could create smarter inventory decisions and ensure products arrive in the right place at the right time.
With the help of IBM Watson’s cognitive intelligence BASF and IBM first built a proof of concept (PoC) to evaluate how AI and machine learning could be utilised to build a more powerful Replenishment Advisor tool. Following the evaluation, IBM Watson and BASF designed a model based on transactional order data and future orders from the company’s ERP system as well as sales pattern reports, volume strategy, inventory levels and shipping times. Using open-source machine learning a custom solution was built to predict future replenishment requirements.
After completing just 10 training cycles the software provided accurate, early warnings for stock replenishment and the optimal time for minimal disruption.
“IBM Cloud and Watson AI services gave us access to a wide range of machine learning models with IBM experience built in, right out of the box,” said Dr. Bernd Lohe, Director Supply Chain Operational Excellence & Digitization at BASF Nutrition & Health. “This meant that we could start analysing our data and training the Replenishment Advisor immediately. The solution also includes data visualizations. During the training phase, this helped our planners to understand system recommendations and to execute effective training loops for machine learning. An integrated chatbot functionality is built into the Replenishment Advisor, allowing staff to interact with the solution using natural language. Based on our successful PoC, we are very satisfied with the IBM Watson portfolio, both in terms of the powerful cognitive capabilities and ease of use.”
KIST Europe: Making factories smarter
KIST Europe – the first overseas branch of Korea Institute of Science and Technology (KIST) – aims to build open innovation platforms for leading Korean and European research institutes and industry partners. One of KIST’s key research areas is the concept of ‘Industry 4.0’, the evolution of technology from centralised systems governed by human intelligence to decentralised machines that can operate independently.
To test and demonstrate the value ‘Industry 4.0’ can add to the manufacturing industry, KIST Europe partnered with SmartFactory and IBM Watson to improve weight measurements, an integral part of quality management. “The technology behind SmartFactory is impressive, but manufacturers are not interested in technology for its own sake. To prove the value of the ‘Industry 4.0’ approach, we need to show how the factory can solve real-world manufacturing problems,” said Marco Hüster, Business Lead AI Implementation at KIST Europe.
In manufacturing, the smallest deviation from the expected weight can signify a fault in either the component, product or production line machinery. As a result, the three companies combined existing SmartFactory technology, data science solutions and a data set of 1,000 real world measurements with machine learning technology to produce a model that can now predict with 98.1% reliable measurement accuracy.
“Weight measurement is a very simple example, but it proves that integrating AI and smart factory technology can have a genuine impact on production-line efficiency and quality management,” commented Dr. Jongwoon Hwang, Group Leader, KIST Europe. “With IBM’s help, we are showing the industry how decentralized AI can help to deliver greater flexibility, optimize process management and predict the performance of production resources. As we continue to move towards a fourth industrial revolution, these capabilities will help pioneering manufacturers transform the industry and create new value for themselves and their customers.”
Amazon
Amazon Web Services (AWS) is Amazon’s comprehensive cloud platform for businesses. A service of AWS is Machine Learning, where customers have the ability to build, train and deploy models, apply and integrated pre-trained AI applications such as recommendations and forecasting, utilise flexible frameworks for custom algorithms and broad compute options as well as harness deep learning technology, analytics and security.
Convoy: Efficiency and the environment
In recent months, Convoy partnered with AWS to utilise its machine learning services developing a solution to make trucking more efficient and environmentally friendly.
40% of miles per year logged by truck drivers are completed with empty trucks. A part of the problem is the industry infrastructure combined with the use of traditional methods. Using AI Convoy looked to automate the process with the help of AWS.
By utilising Amazon SageMaker, Convoy developed a machine learning model that can analyse millions of shipping jobs and trucker availability, resulting in recommended matches that are cost and time efficient. In addition to this, the model will also recommend matches for the journey back, which reduces the number of miles completed by empty trucks, which in turn will have a positive impact on the environment.
“As we work with more shippers and carriers, we get a better understanding of how much capacity is available and how much demand is coming in on specific lanes,” says Casey Olives, Head of Data Science at Convoy. “Being able to have a contextual view of the entire network will enable us to drive efficiencies in utilisation and costs, benefiting both carriers and shippers.”
TuSimple: Autonomous Trucks
TuSimple, one of the world’s largest self-driving truck companies, has partnered with AWS to develop autonomous vehicles. Built primarily using the Apache MXNet deep learning framework on AWS, TuSimple vehicles have built in servers loaded with up to 100 different AI modules. These modules distinguish the types of cars on the road and the speed of other objects around the truck providing a steady stream of data from cameras, LiDAR, and radar equipment to build a live 3D model of the road that is constantly updated as the truck moves. After completing a successful delivery, the results are updated to the modules on every trucks server after completing safety test and simulations to ensure it will behave as expected. With the help of AWS’s massive computing power, this process takes hours rather than weeks.
Currently TuSimple’s trucks are at a ‘level 4’ autonomous vehicle classification and have a 5cm accuracy at 65 mph with a loaded trailer. By 2020, Xiaodi Hou, president and CTO of TuSimple, wants to remove human ‘fail-safes’ from the vehicles.
With firms worldwide at the beginning of an exciting future in the leveraging of new technology in the supply chain space, machine learning is set to feature even more prominently in company’s operations over the coming years.
For more information on all topics for Procurement, Supply Chain & Logistics - please take a look at the latest edition of Supply Chain Digital magazine.
- The Art of Supply Chain Planning with Gartner, SAP, KinaxisSupply Chain Risk Management
- How to Boost Supply Chain Visibility with Tive and ArvatoSupply Chain Risk Management
- Top 100 Women 2024: Stephanie Rankin Smith – No. 8Operations
- Why SAP Supply Chain Solutions Could Transform ManufacturingTechnology